Affiliate links on Android Authority may earn us a commission. Learn more.
How machine learning protects your wallet and identity
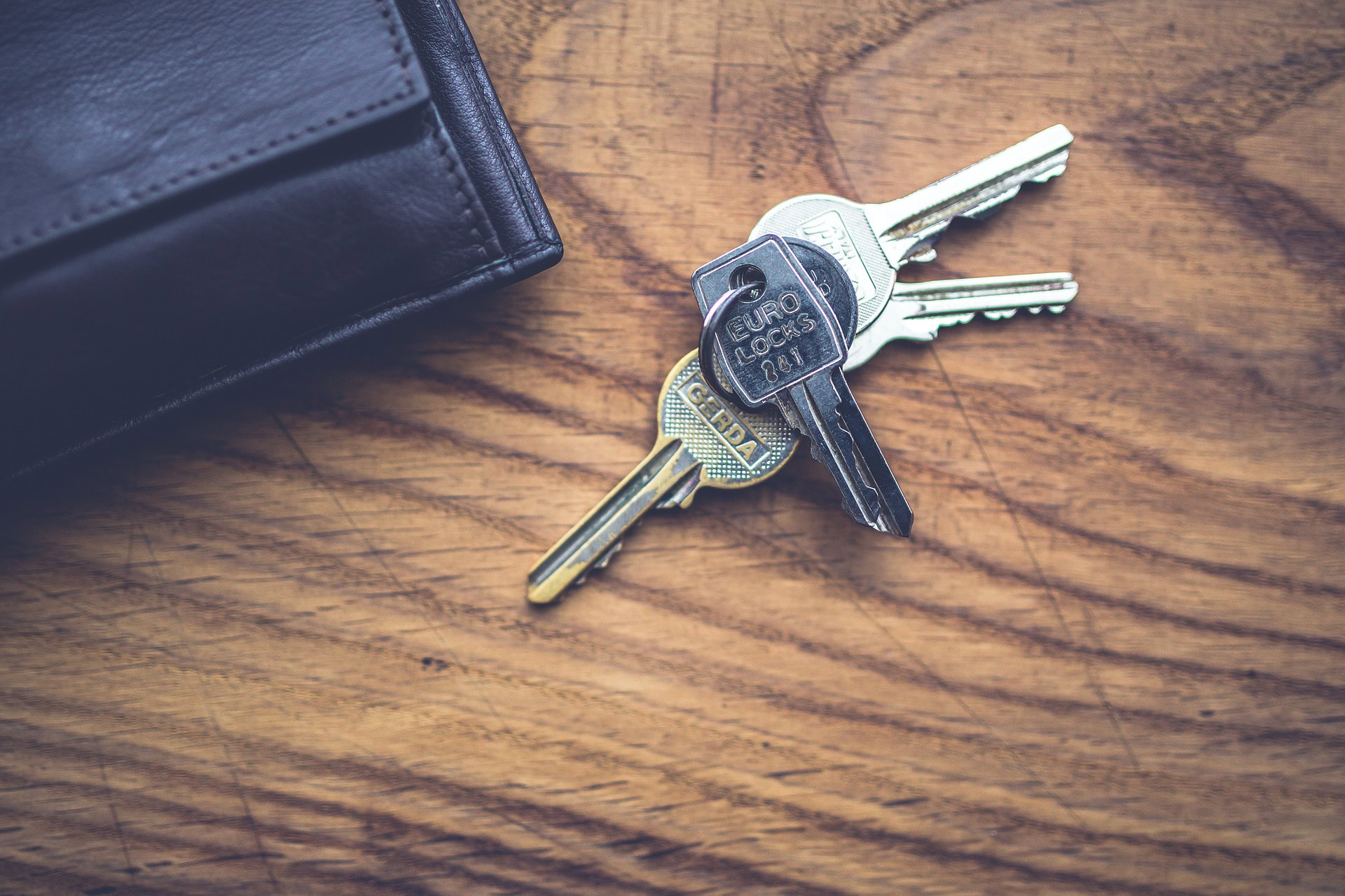
The progress of technology and its impact in our lives is marked by fundamental shifts in direction and capabilities that eclipse all that came before it. For example, the arrival of the Web changed the way we communicate, work and play, while obliterating the bulletin board systems that preceded it. Likewise, personal computers overshadowed the mainframes that came before them and most recently smartphones have risen to take the place of cellphones, digital cameras, camcorders and MP3 players.
We are on the verge of a new shift, a new era for computing. This one won’t reach its peak as quickly as the previous eras, but it will go further than anything that has come before it. What is this new tech? Machine learning and AI.
Before you start quoting lines from The Terminator and worrying about the end of life as we know it, let’s clarify the terms machine learning and AI. Machine learning is about making systems that can learn from experience. By showing a machine thousands of kitten photos then it learns what is a kitten and it can distinguish between a kitten and a puppy.
The goals of artificial intelligence are much broader. AI researchers are trying to create a machine which can mimic a human mind. While ML is a subset of AI, it shouldn’t be thought of as less important.
While developing machine learning systems is hard (and general AI is even harder) you have probably already used machine learning technology, even if you didn’t know it. For example, if you have used any of the popular music streaming services then the songs you like have probably been used by a machine learning algorithm on a server to try and find new music that you will like.
But with all this data being used and analysed there are also dangers. Risks of security breaches, hacking, cyber criminals, unfriendly nation states and more. These risks are not just technical, but they present a risk to people, families and society. Technology companies have a responsibility to society that is greater than their need to sell products. In many ways tech OEMs are the inventors of the future but they are also the guardians of our privacy, security and safety.
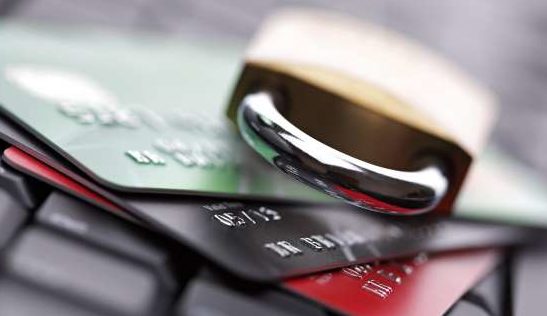
Beyond the server room
Once machine learning had established itself in the server room, it moved out in search of new territory. One such pasture is mobile, with a growing prevalence of machine learning on mobile related news. Google with its shift from “mobile-first to AI-first”, the emergence of popular digital assistants, and a new breed of smartphone which emphasize their ML pedigree including the MATE 10 with its NPU sporting Kirin 970 and Google’s revelation that the Pixel 2 includes new special hardware for image processing and ML.
But there is more to ML than just kittens. If a smartphone or a smart IoT device has ML capabilities then it is able to use those capabilities for a multitude of tasks including for security, privacy and fraud prevention.
By learning patterns about times, places, accelerometer readings (i.e. how you hold and move your phone), amounts and online habits, then a machine learning algorithm will be able to help protect a user from cyber criminals. For example, ML technology could halt the authorization for a NFC payment when the phone is upside down in a pocket.
When it comes to ML applications in security, the possibilities are endless
The possibilities are endless. Consider smart firewalls or smart malware scanners that incorporate patterns learnt from the device’s owner and not just some standard rules shipped from the factory.
Likewise the behavior of IoT devices can be monitored and patterns learned. When an IoT device starts behaving outside of its norms (because it has been hacked) then it can be isolated or quarantined.
These advances in device security and fraud protection need more than just a technical solution, they need a commitment from the tech companies themselves to ensure that they embrace their responsibilities and make security a primary design consideration for all devices. To that end it is good to see Arm’s recent launch of its Security Manifesto and its efforts to make tech companies understand their social responsibilities in the digital age.
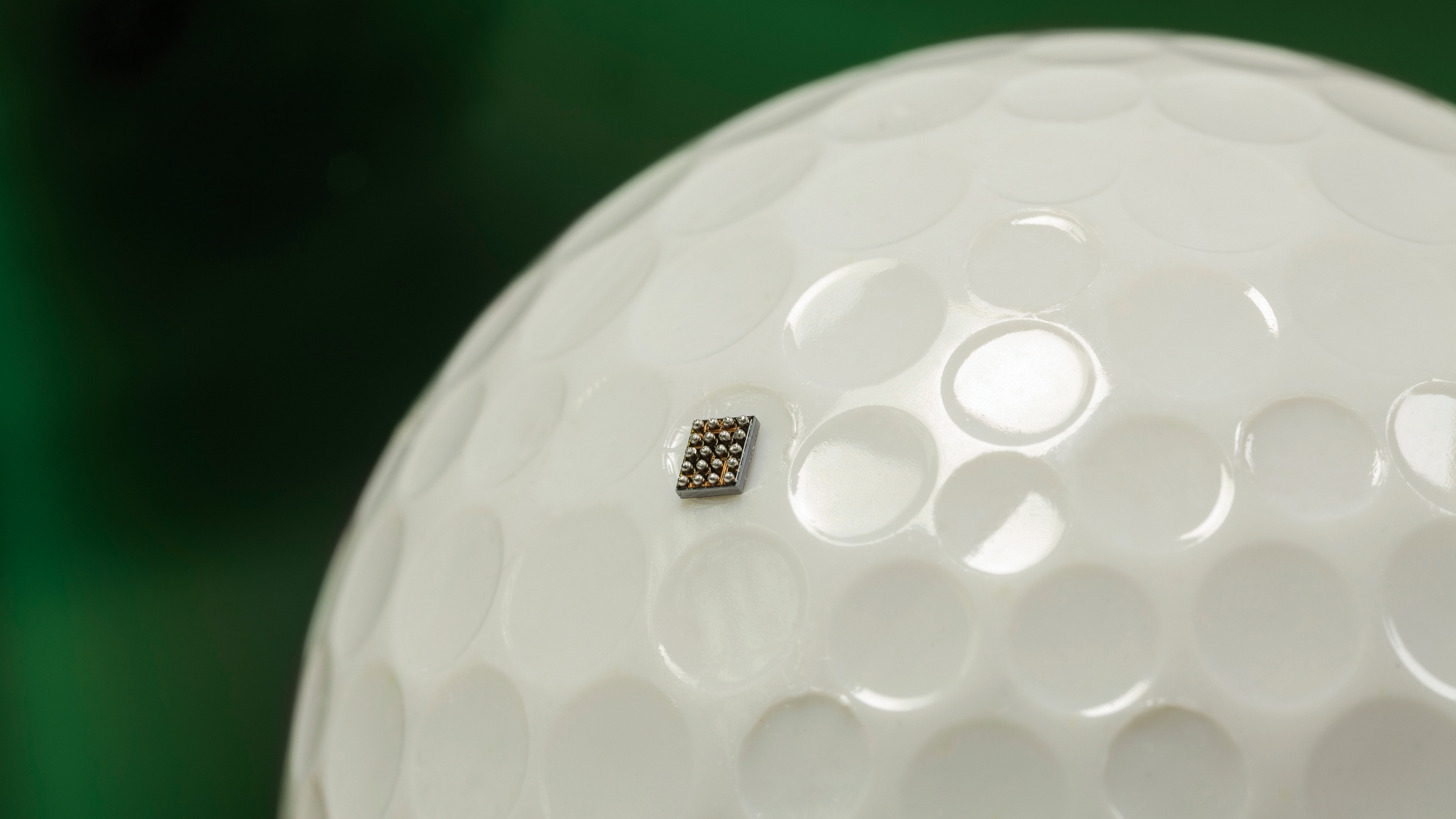
Beyond devices
Outside of consumer devices there are huge strides being made in other areas like self driving and automation. Machine learning is being used as the tool to tackle many of the problems that were previously thought of as unsolvable.
One thing that binds all these different machine learning solutions together is the ubiquitous use of Arm processors. From self-driving cars to smartphones with machine learning capabilities, Arm processors are central. Arm technology has become the de-facto standard for many areas, especially where power efficiency, rather than outright CPU cycles, is more important.
Machine learning is a tool that can help solve problems that were previously thought of as unsolvable
Arm’s business model allows silicon vendors to create custom solutions for a wide number of markets and include ML capabilities as needed. Looking at mobile we see HUAWEI using Arm-designed CPU cores and an Arm-designed GPU together with its NPU components to create devices with offline ML abilities. The same can be said for self-driving cars or for the automation industry. For ML tech to fully achieve its potential, OEMs need a flexible and power-efficient platform, a platform that ARM is providing.
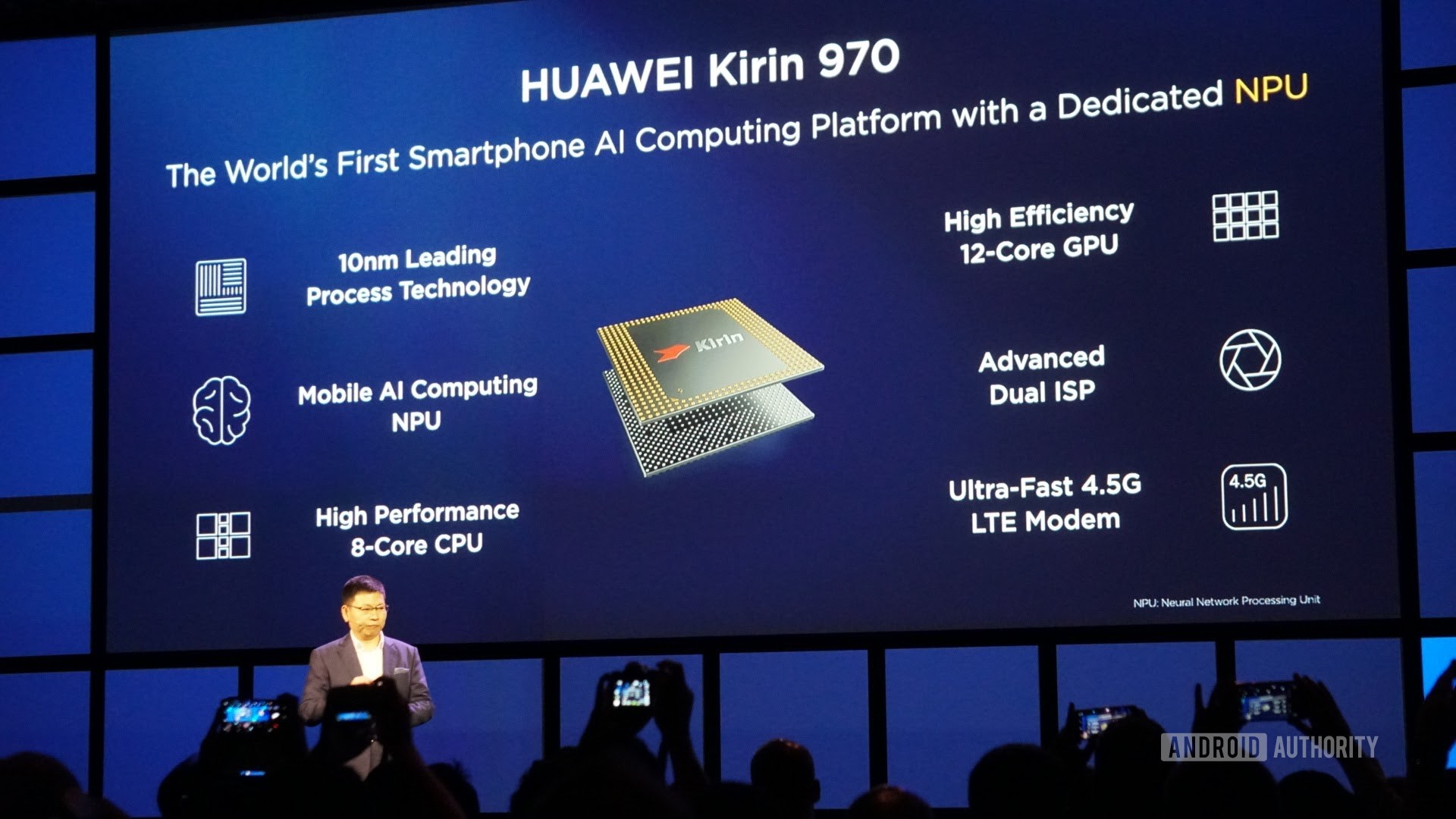
Offline ML abilities aren’t the norm right now, in fact the real power of ML will come from the distributed intelligence that is deployed from devices through to the cloud. The power of group learning far outweighs the abilities of individual learning. When people drive there is normally just one set of eyes on the road, but we have all had moments where a passenger has alerted us to a possible danger. Now imagine machine learning where every car can share information about road conditions or obstacles, or every device can share its experience from within its domain.
The real power of ML will come from the distributed intelligence that is deployed from devices through to the cloud
This means that AI doesn’t happen in just one place, it happens at different points from the devices to the cloud, with each layer adding to what has already been processed.
Wrap-up
Machine learning is already helping us in many ways and this is only the beginning. As ML techniques improve and as our understanding of what can be achieved increases, then the effects of ML in our daily lives will also rise. This comes with its own challenges and while companies like Arm can provide the technology, they can also provide the guidance to make sure it gets done right without putting consumers at risk from sloppy practices and half-baked security solutions.