Affiliate links on Android Authority may earn us a commission. Learn more.
The complexities of ethics and AI
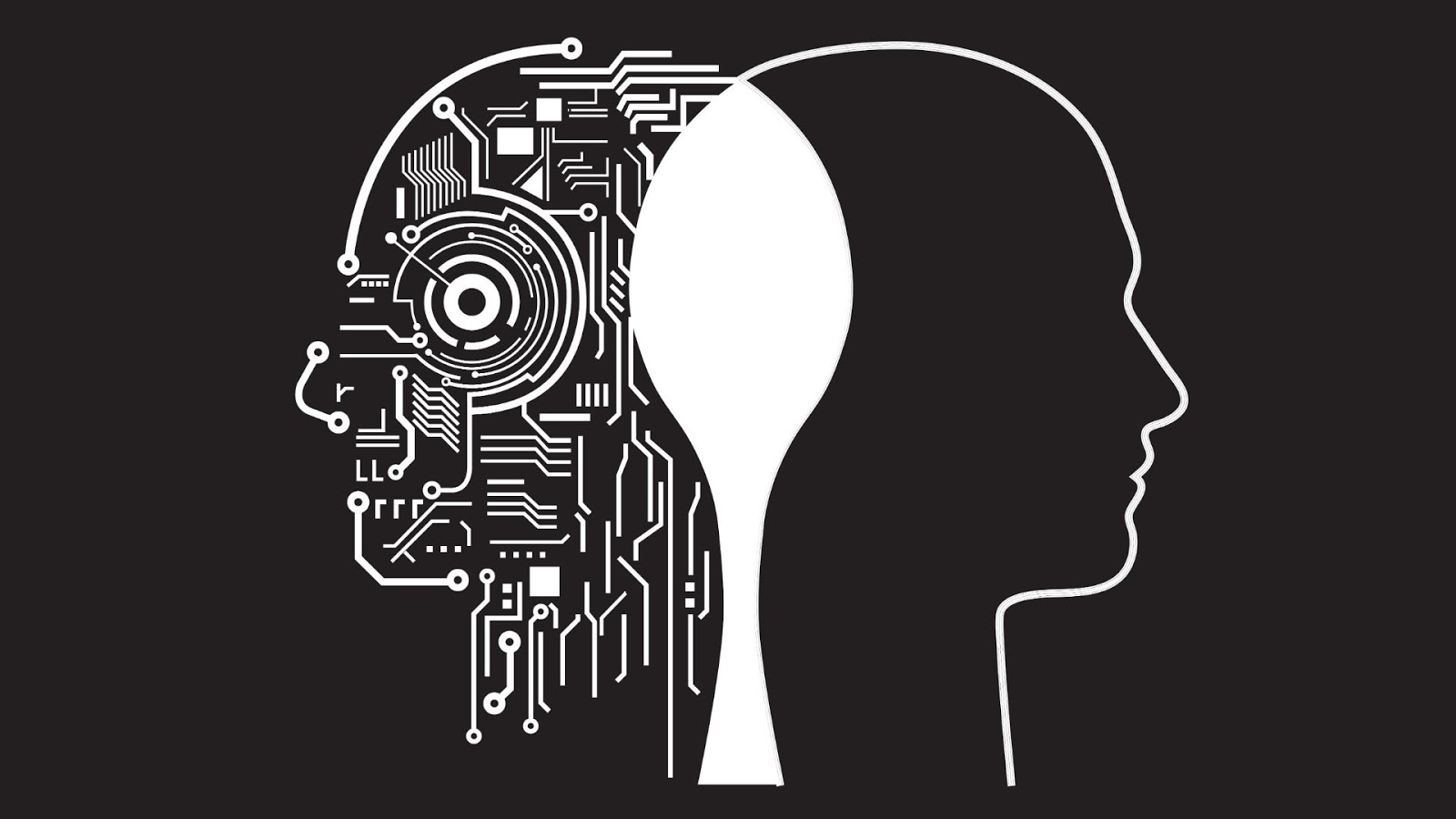
The starting point for any discussion about AI will almost certainly focus on how we should use it, and the advantages and disadvantages it could bring. Google’s Sundar Pichai recently suggested AI could be used to help solve human problems — a noble goal. How we use it to solve these problems, and ultimately how successfully they will be, is going to depend on our ethics.
A machine learning algorithm can’t tell you whether a decision is ethical or not. It’s going to be up to human creators to imbue machines with our own sense of ethics, but it’s not so easy just code in the difference between right and wrong.
Teaching ethical subtleties
Today’s machine learning and artificial intelligence algorithms have become very good at sifting through huge chunks of data, but teaching machines to interpret and use that data can quickly lead to some ethical problems.
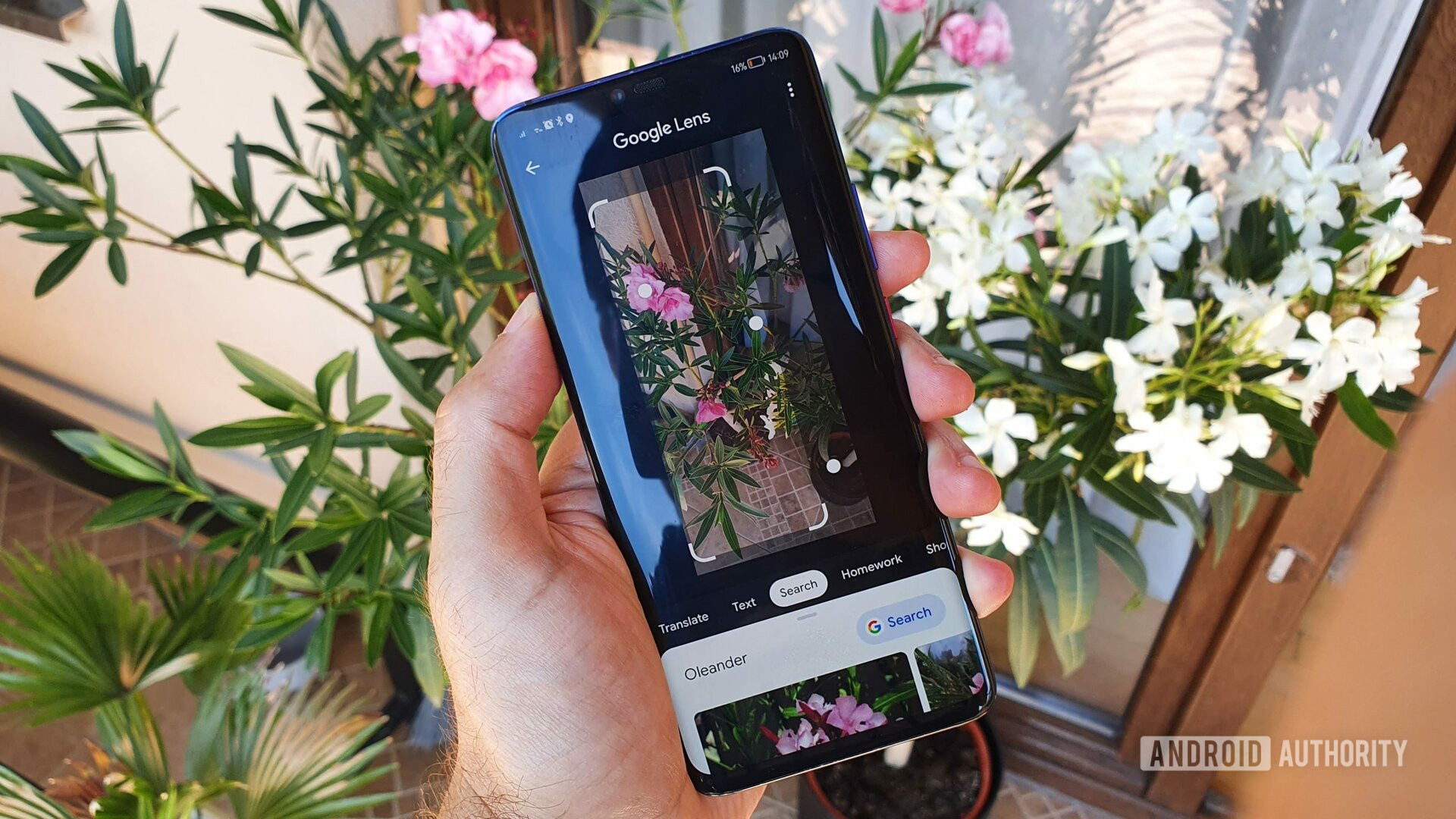
Consider the very useful application of using AI to manage limited emergency resources around a city. As well as calculating the fastest possible response times and balancing incident priorities, the system will also have to reassess priorities on the fly and potentially reroute resources, creating the need for some more contextual and ethical decision making.
Maximizing the number of people helped seems like a reasonable goal for the AI, but a machine might attempt to cheat by over-resourcing relatively low-risk emergencies to maximize its score and neglect incidents with a lower probability of success. Tightening up those priorities could also lead to the opposite problem of paralysis, where the system continually redirects resources to new high priority cases but never gets around to solving lower priority ones. The AI would need to take into account the severity or subtleties of every incident.
By what metric do you decide the difference between sending resources to a small fire that threatens to spread or a car accident? Should resources be diverted from helping a minor case close by which has been waiting awhile to attend a new, more serious incident further away? These are tough questions for even a human to decide. Programming exactly how we want AI to respond could be even harder.
The field of AI safety is trying to anticipate unintended consequences of rules — defining workable reward or goal systems — and prevent AI from taking shortcuts. Everyone has slightly different ethical ideals, and how AI handles these situations will almost certainly be a reflection of the ethical values we pre-program in them (but more on that later).
The field of AI safety is grappling with anticipating unintended consequences of ethical rules, defining workable reward/goal systems, and preventing AI from taking shortcuts to achieve these goals.
It’s not all doom and gloom though, some believe we may be able to achieve better results in some of the world’s less desirable situations by making use of AI to apply ethical rules more consistently than humans do. Observers are often fearful about the potential of autonomous weapons, not just because they’re lethal but also because they might remove the human attachment to the moral implications of war.
Most people abhor violence and war, but autonomous weapons present designers with the unique opportunity to install ethical rules of engagement and treatment of civilians without worrying about the momentary lapses during adrenaline-fueled situations. Coming up with set rules applicable across every possible combat situation would be very difficult though.
A more feasible solution could be to use machine learning to come to its own conclusions about ethics and adapting them to unpredictable situations by programming core principles and relying on iterative learning and experience to guide the results. Conclusions are less predictable this way and really depend on the type of starting rules provided.
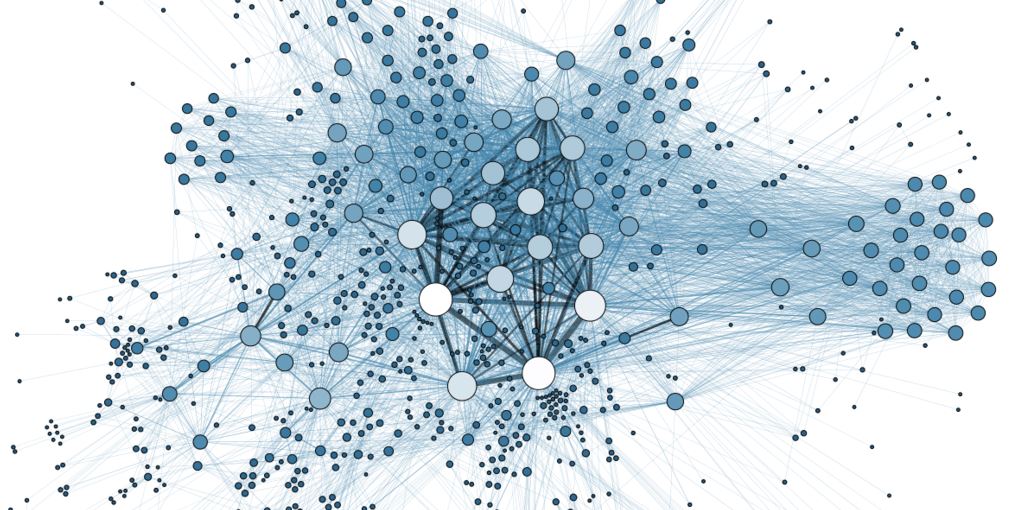
Bias and discrimination
Given that human input and judgements are inevitably going to shape AI decision making, data modeling, and ethics, extra care is required to make sure our biases and unfair data don’t make their way into machine learning and AI algorithms.
Some popular examples have already highlighted potential issues with biased data or more questionable applications of machine learning. Algorithms have incorrectly overemphasized recidivism rates for black convicts. Image learning has reinforced stereotypical views of women. Google had its infamous “gorillas” incident.
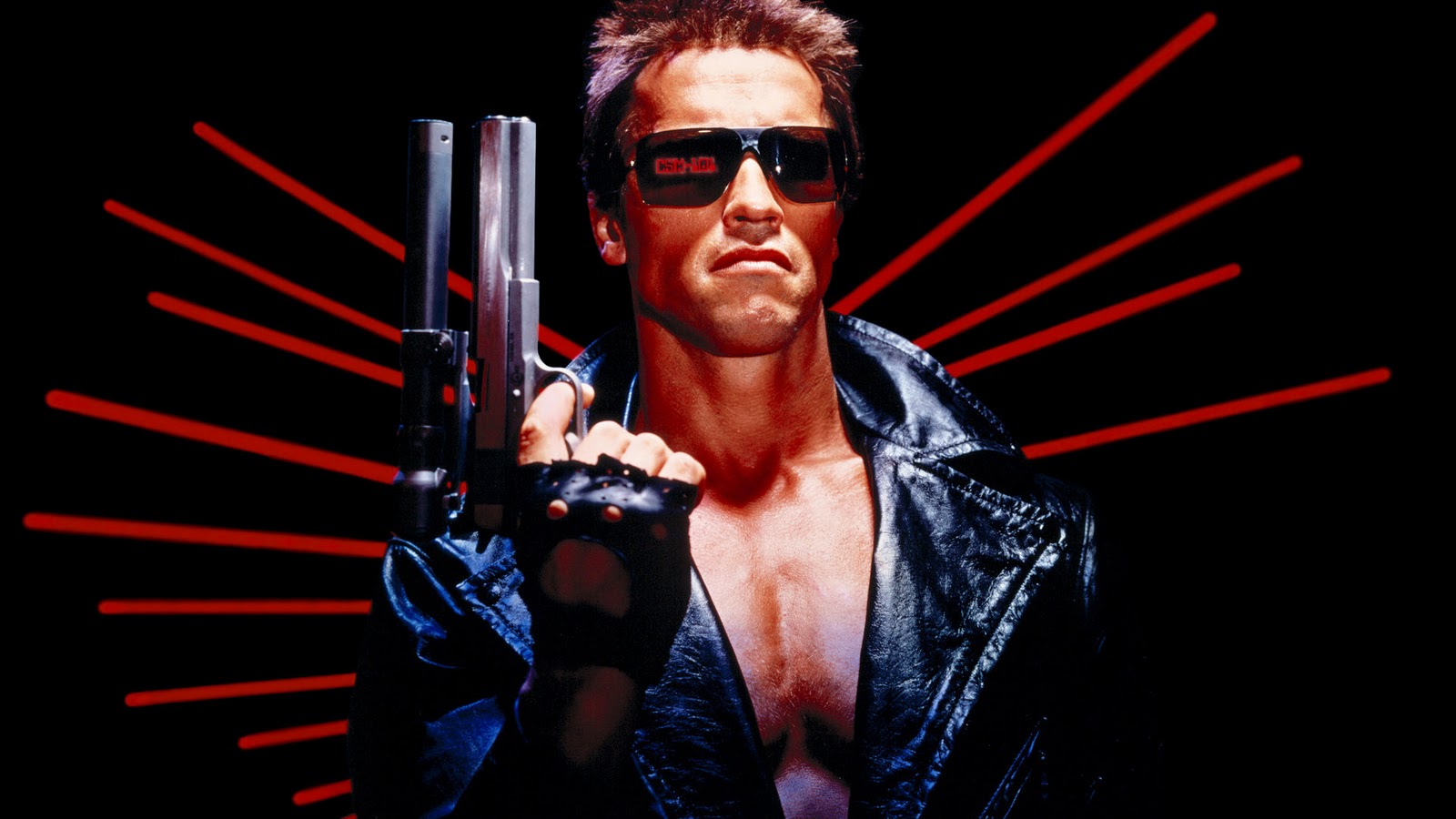
In any given data set, there are perfectly valid reasons for some biases to exist. Gender, race, language, marital status, location, age, education, and more can be valid predictors in certain situations, though often as just part of a multivariate analysis. Potential problems arise when algorithms attempt to exploit particularly subjective data to take shortcuts — emphasizing a stereotype or average at the expense of broader factors — or when the data collection is fundamentally flawed.
An algorithm designed to hire the most suitable candidates for a job may identify trends based along sex in the industry, such as the higher representation of women in teaching or men in engineering. On it’s own this observation isn’t harmful — it can be useful for planning around employee needs. If the algorithm placed undue emphasis on this attribute in an attempt to maximize its hiring rate, it could instantly discard applications from the minority sex in the industry. That’s not helpful if you’re looking to hire the best and brightest, and it also reinforces stereotypes. The goals and ethics of an AI system have to be clearly defined to avoid such issues, and big companies like IBM are attempting to solve these problems by defining and scoring ethical AI systems.
Machine learning can't tell you whether a decision is ethical or not. An algorithm is only as ethical as the data and goals fed into it.
But if a system produces results we find to be unethical, do we blame the algorithm or the people who created it? In instances where an individual or group has built a system with deliberately unethical goals, or even in cases where sufficient attention hasn’t been paid to the potential results, it’s reasonably straightforward to trace back responsibility to the creators. After all, an algorithm is only as ethical as the data and goals it’s fed.
It’s not always crystal clear if blame can be attributed to the creators just because we don’t like the outcome. Biases in data or application aren’t always obvious or even possible to definitively identify, making a hindsight approach to responsibility more of a gray area. It can be difficult to trace how an AI comes to a conclusion even when its machine learning approach is based on a simple set of ethical rules. If AI is empowered to adapt its own ethics, it’s much harder to blame the creators for undesired consequences.
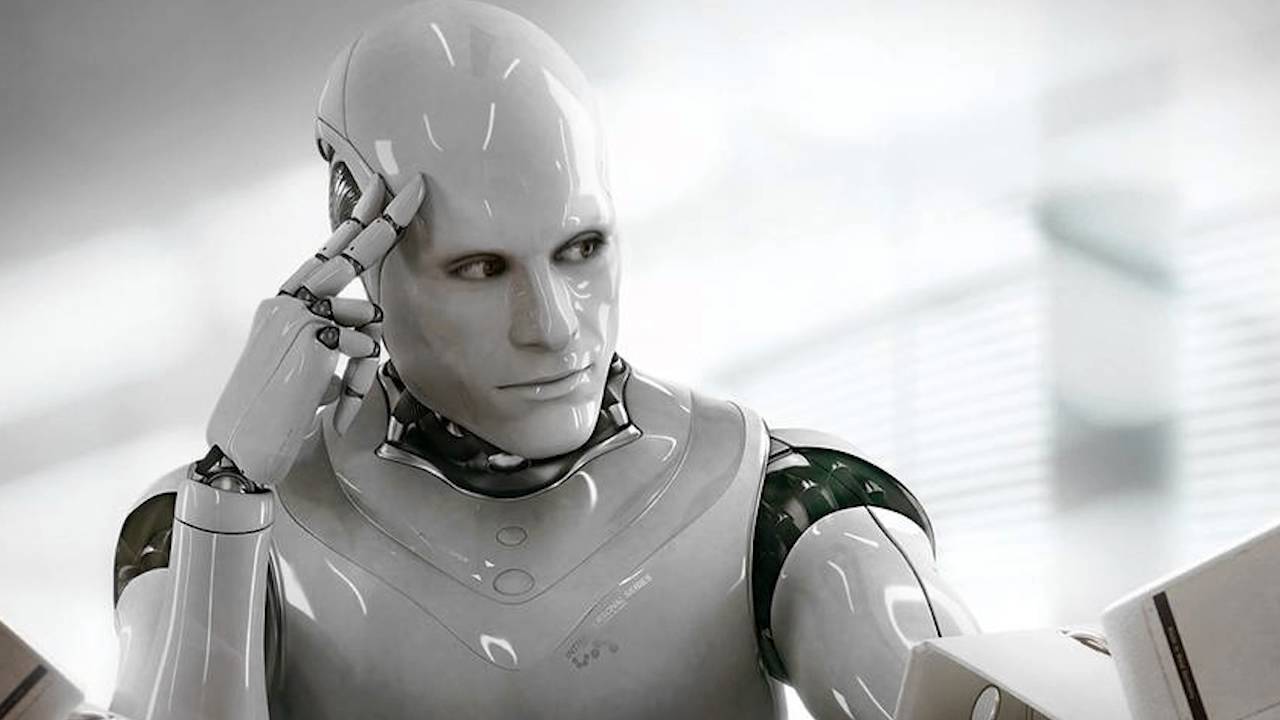
Machines that can think
We may eventually have to deal with the other side of the coin, too: how should humans treat machines that can think?
There’s still a major debate to be had about the attributes general artificial intelligence would have to possess in order to qualify for genuine original thought, or human-like intelligence, rather than a very compelling illusion. There’s already a consensus on the key differences between narrow (or applied) and general AI, but the jury is still out regarding how to define and test “true” artificial intelligence.
It’s no understatement to say that navigating AI ethics, its implementation, and implications, is a minefield.
The implications for such a discovery or broader definition of intelligence could force us to assess how we treat and view AI. If AI can truly think, should it be afforded the same or different legal rights and responsibilities as humans? Can AI be held accountable for a crime? Would it be immoral to reprogram or switch off self-aware machines? We’re barely scratching the surface of the potential ethical and moral problems AI might present.
Suggested criteria for assessing AI intelligence includes complex scenario comprehension, making decisions based on partial information, or an agent’s general ability to achieve its goals in a wide range of environments. Even these don’t necessarily satisfy those looking for a definitive difference between intelligence and a state machine. The other part of the problem is that cognitive and neuroscientists are still picking apart attributes of the brain related to the human ability to think, learn, and form self-aware consciousness. Defining intelligence — not just for AI but also for humans — is perhaps one of the greatest unsolved questions of our time.
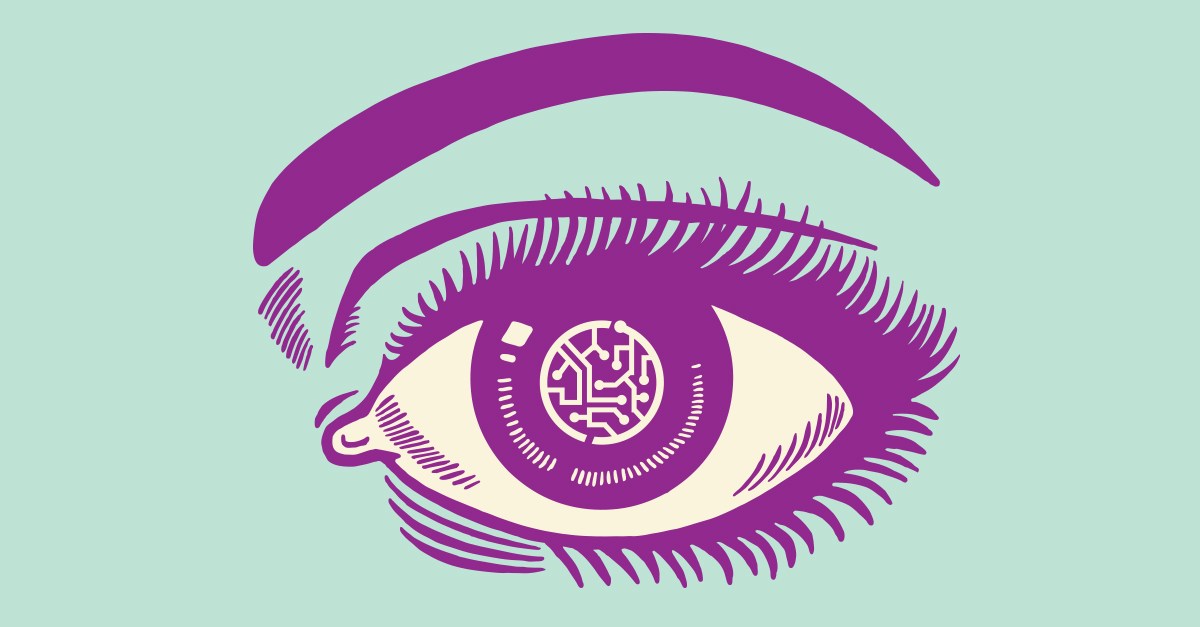
Wrap up
AI ethics encompass a huge range of topics and scenarios, ranging all the way from how we should use it, to biases and creator accountability, to the very nature of how we should value and treat all types of intelligence. The accelerating pace of AI development and use in our everyday lives makes coming to grips with these topics an urgent necessity.
It’s no understatement to say figuring out AI ethics, its implementation, and implications, will be a minefield. It can be done, but it’s going to require some very thorough discussions and consensus building across the industry and perhaps between governments too.