Affiliate links on Android Authority may earn us a commission. Learn more.
AMD hints at how RDNA could beat Qualcomm's Adreno GPU
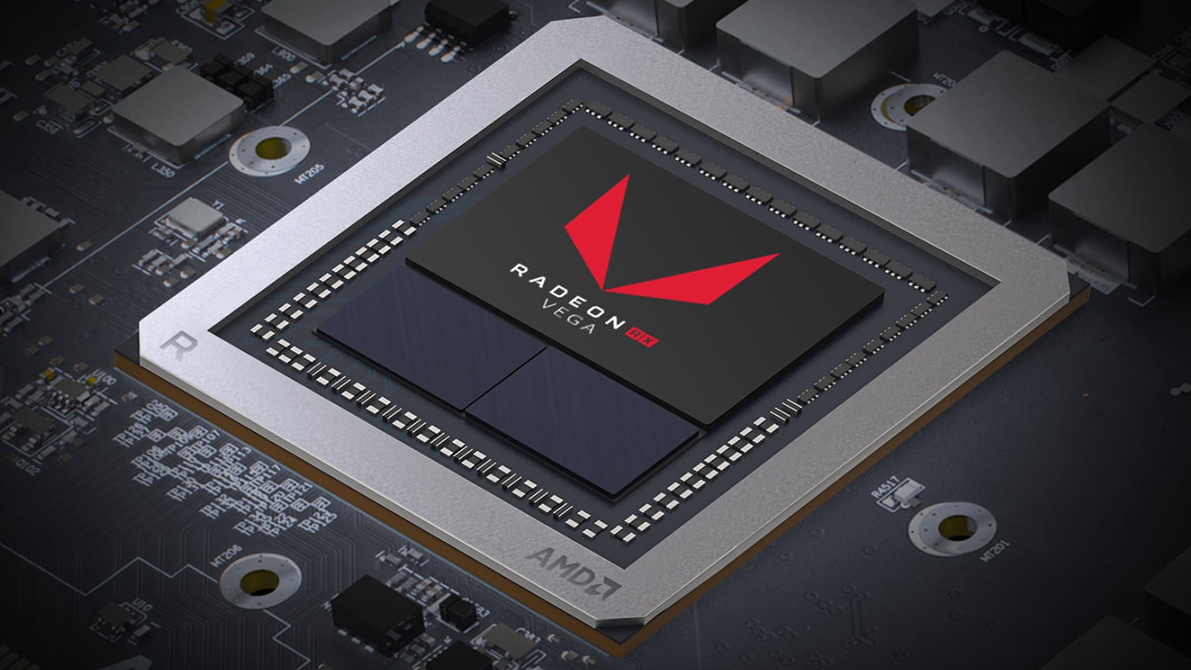
Back in June, Samsung and AMD announced a strategic partnership to bring AMD’s “Next Gen” GPU architecture to mobile devices. More recently, AMD has published a whitepaper on its latest RDNA microarchitecture. The paper reveals plenty about how AMD’s high-end RX 5700 graphics card works and alludes to future low-power designs too.
By graphics microarchitecture, we mean the fundamental building blocks that make a GPU work. From the small number crunching cores through to the memory and connections that tie everything together. RDNA encompasses the instructions and hardware building blocks used inside AMD’s latest GPUs for PCs, next-generation gaming consoles, and other markets.
Before we dive in, there’s nothing in the paper about Samsung’s upcoming GPU. That won’t launch until 2021 at the earliest and will almost certainly be based on Navi’s successor and the next iteration of RDNA. However, there is some juicy information on the architecture that we can interpret for future mobile devices.
GPUs built on the RDNA architecture will span from power-efficient notebooks and smartphones to some of the world’s largest supercomputers.AMD's RDNA Whitepaper
Can AMD really scale to Samsung’s needs?
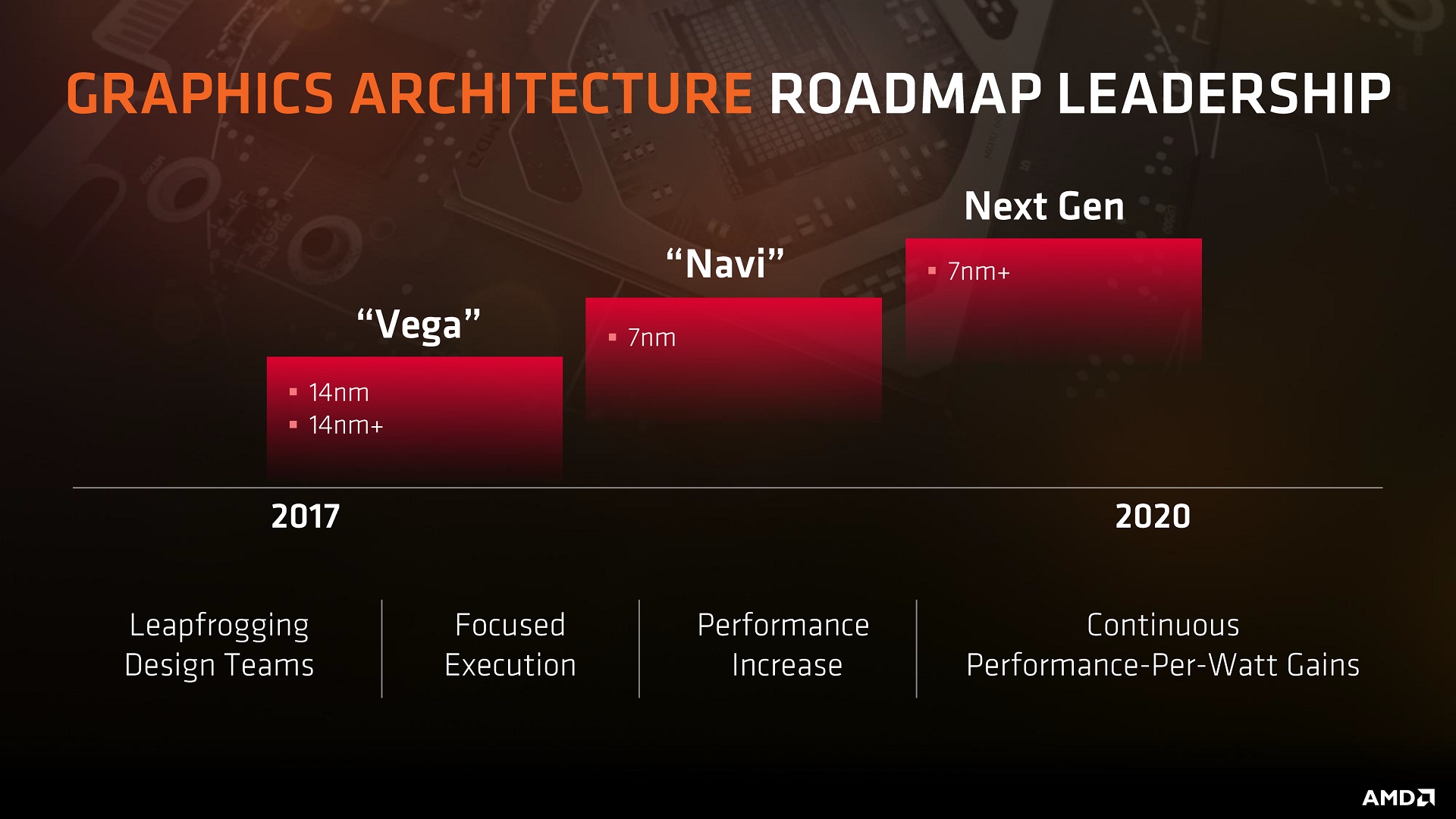
Before we get to the technical stuff, it’s worth asking what aspects of AMD’s graphics architecture appeal to a mobile chip designer like Samsung, especially given that Arm and Imagination offer optimized, tried-and-tested mobile graphics products. Ignoring licensing arrangements and costs, for now, let’s focus on what AMD’s hardware offers Samsung.
We can’t say a lot about performance potential in a mobile form factor from the whitepaper. But we can see where RDNA offers optimizations that might suit mobile applications. The introduction of an L1 cache, shared between the Dual Compute Units (the math crunching parts), reduces power consumption thanks to fewer external memory reads and writes. The shared L2 cache is also configurable from 64KB-512KB slices depending on the application’s performance, power, and silicon area targets. In other words, the cache size can be tailored to a mobile performance and cost point.
Improved energy efficiency is a key part of the changes to RDNA.
AMD’s architecture also moves from 64 work-items with GCN to supporting narrower 32 work-items as well with RDNA. In other words, workloads compute in parallel operations 32 at a time in each core. AMD says this benefits parallelism by distributing workloads to more cores, improving performance and efficiency. This is also better suited to bandwidth-limited scenarios like mobile, as moving big chunks of data around is energy-intensive.
At the very least, AMD is paying plenty of attention to memory and power consumption — two critical parts in any successful smartphone GPU.
Radeon excels at compute workloads
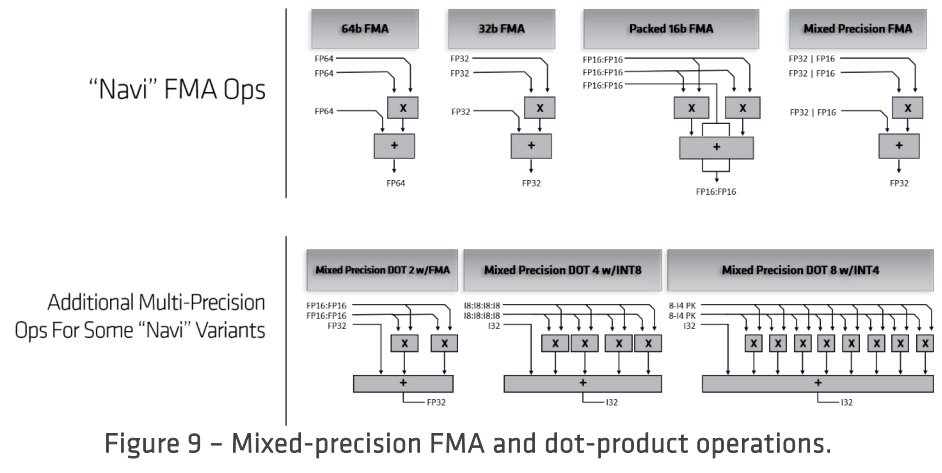
AMD’s Graphics Core Next (GCN) architecture, the precursor to RDNA, is also particularly strong at machine learning (ML) workloads. AI, as we know, is now a big deal in smartphone processors and is only likely to become more common over the next five years.
RDNA retains high-performance machine learning credentials, with support for 64, 32, 16, 8, and even 4-bit integer math in parallel. RDNA’s Vector ALUs are twice as wide as the previous generation, for faster number crunching and also perform fused multiply-accumulate (FMA) operations with less power consumption than previous generations. FMA math is common in machine learning applications, so much so that there’s a dedicated hardware block for it inside Arm’s Mali-G77.
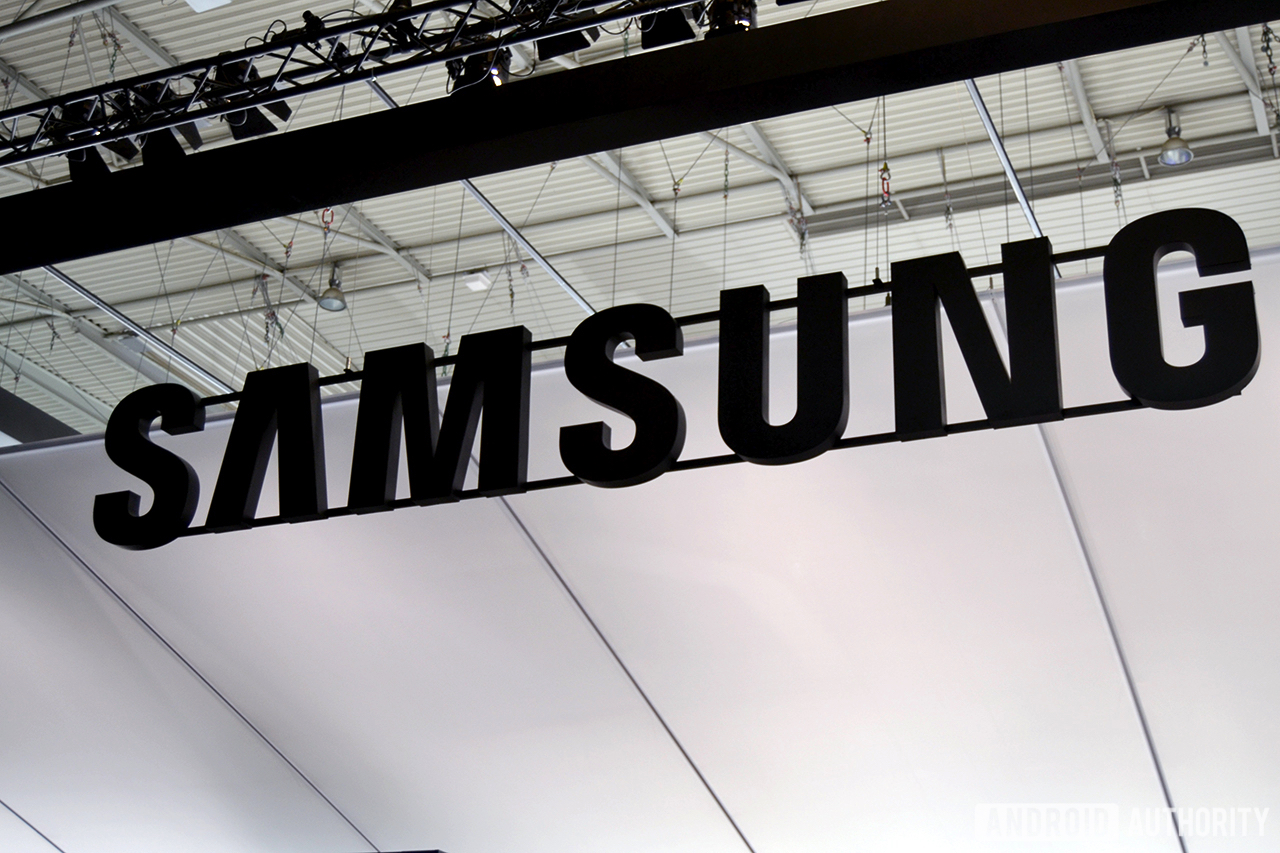
Furthermore, RDNA introduces Asynchronous Compute Tunneling (ACE) which manages compute shader workloads. AMD states that this “enables compute and graphics workloads to co-exist harmoniously on GPUs.” In other words, RDNA is much more efficient at handling ML and graphics workloads in parallel, perhaps lessening the need for dedicated AI silicon.
I don’t want to make any performance projections based on a document that primarily talks about the desktop-class RX 5700. Suffice to say that, feature-wise, RDNA certainly looks appealing if you want to utilize silicon space for graphics and ML workloads. Furthermore, AMD is promising more performance-per-watt gains to come with 7nm+ and its upcoming “Next Gen” implementation of RDNA, which is what Samsung will be using.
RDNA: Designed to be flexible
In addition to the above, there’s plenty of technical information on the new narrower wave32 wavefronts, instruction issuing, and execution units in the paper if you’re curious. But the bit that’s most interesting from my perspective is RDNA’s new Shader Engine and Shaders Arrays.
To quote from the white paper directly: “To scale performance from the low-end to the high-end, different GPUs can increase the number of shader arrays and also alter the balance of resources within each shader array.” So depending on your target platform, the number of Dual Compute Units, the size of the L1 and L2 caches, and even the number of render backends (RBs) change.
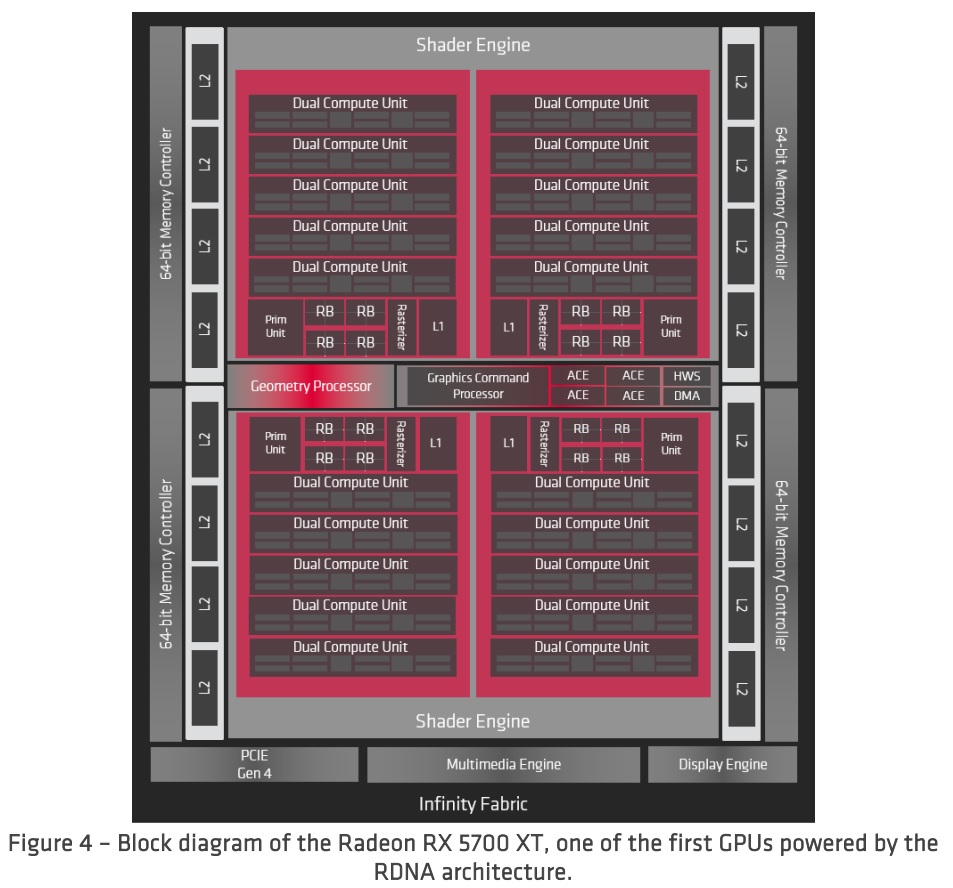
AMD’s previous GCN architecture already offered flexibility in the number of computing units to build GPUs at different performance levels. NVIDIA does the same thing with its CUDA core SMX groups. NVIDIA’s Tegra K1 mobile SoC used just one SMX core to fit into a tiny power budget, and AMD scales its core count to build more efficient laptop GPUs. Likewise, Arm Mali GPU cores scale up and down in number depending on the required performance and power targets.
RDNA is different though. It provides more flexibility to tweak performance and therefore power consumption within each Shader Array. Rather than just adjusting the compute unit count, Samsung, for example, can experiment with the number of arrays and RBs, and the amount of cache too. The result is a more flexible platform optimized design that should scale much better than previous AMD products. Although what sort of performance can be obtained within the constraints of a smartphone remains to be seen.
RDNA shader 'cores' for mobile will be different from cores used in desktop and server products.
Samsung’s AMD GPU in 2021
According to Samsung’s latest earnings call, we’re still “two years down the road” from the launch of the company’s RDNA-based GPU. This suggests a 2021 appearance. In that time, it’s likely that there will be further tweaks and changes to the architecture behind the RX 5700, particularly as AMD further optimizes power consumption.
However, the building blocks for RDNA detailed in the whitepaper give us an early peek on how AMD plans to bring its GPU architecture to low power devices and smartphones. The key points are a more efficient architecture, optimized mixed-compute workloads, and a highly flexible “core” design to suit a wider range of applications.
AMD GPUs aren’t the most power-efficient in the PC market, so it’s still surprising to hear ambitions ranging from servers to smartphones with a single architecture. It will certainly be interesting to dive deeper into Samsung’s implementation of RDNA come 2021.