Affiliate links on Android Authority may earn us a commission. Learn more.
What’s next for machine learning?
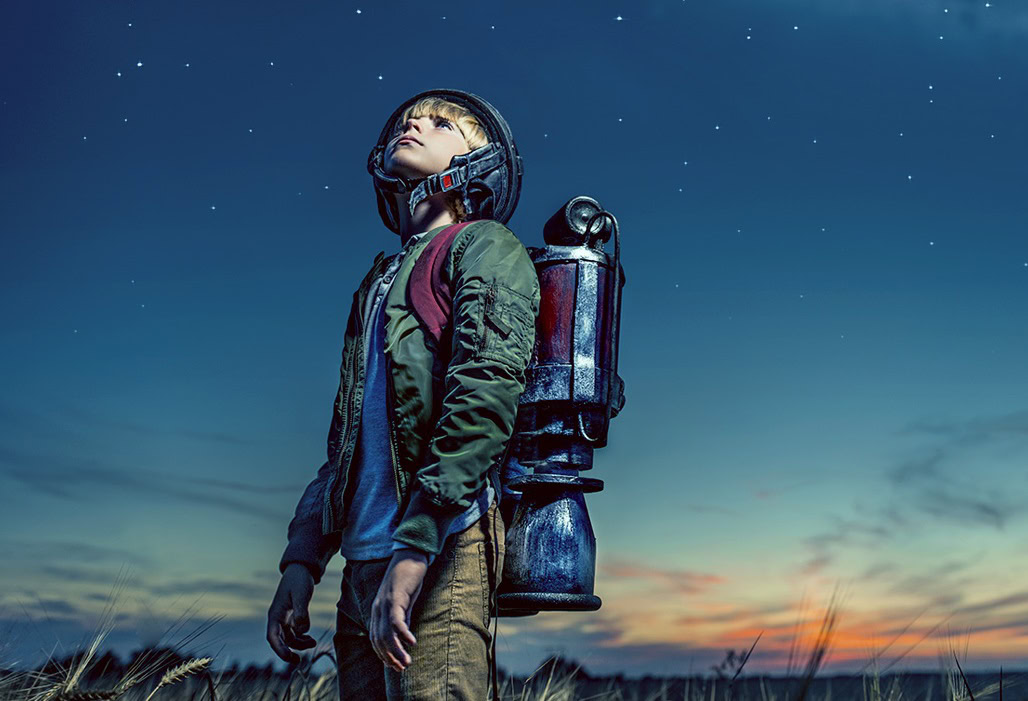
What is the single greatest adaptation of the human species?
Definitely not our impressive physique, woolly coats, or prodigious olfactory capabilities. We kind of suck at all of those. Our greatest trait is pattern recognition. In fact, it’s so strong that we often read patterns where none exist. (See: astrology.)
Historically, our ability to recognize patterns let us deduce when danger was near in time to take action. It also let us develop languages more complicated than a series of grunts and associations. You could even say it’s the foundation of modern science.
Rise of the Machines
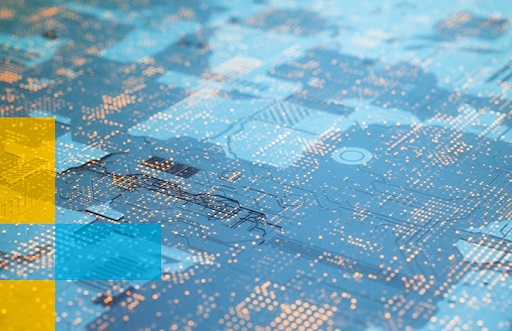
In ye olde times, machines were notoriously bad at pattern recognition — they could really only follow a set of pre-programmed instructions. The rise of machine learning has yielded systems and devices that can actually interpret data and use it to improve themselves.
Machine learning already touches nearly every aspect of our lives, changing them for the better. As good as we are at detecting patterns, machines are far, far better at it – and this pattern detection comes in pretty handy in a huge range of ways, from speech recognition to stock market anticipation.
So what can we expect from this field in 2019?
Making the Digital Physical
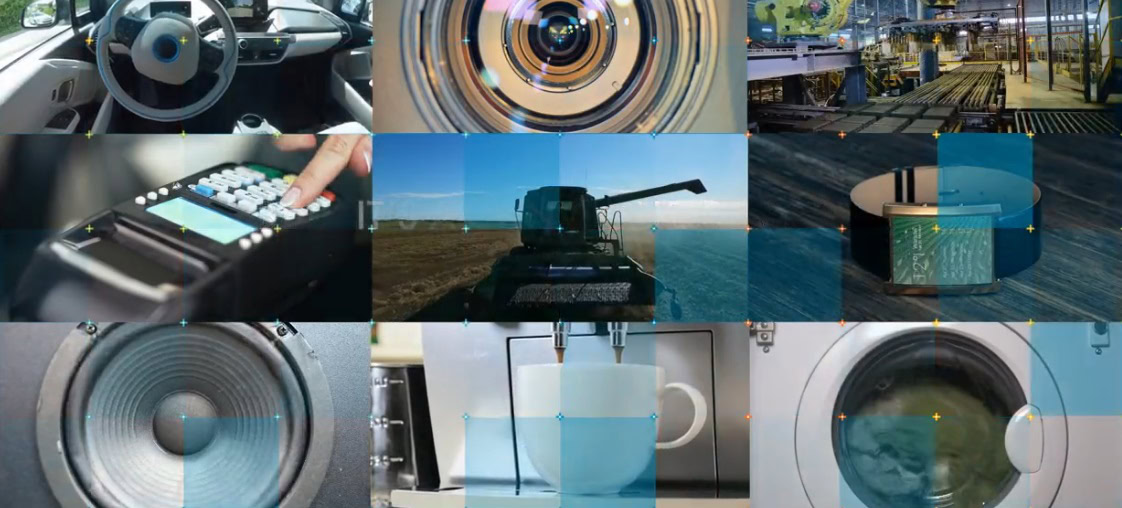
Companies heavily invested in both machine learning and small-scale computing are clearing the path for the future of ML. Arm is at the forefront of this effort. Its technology is improving everything from first-response medical care to snapping selfies.
Consider Corti
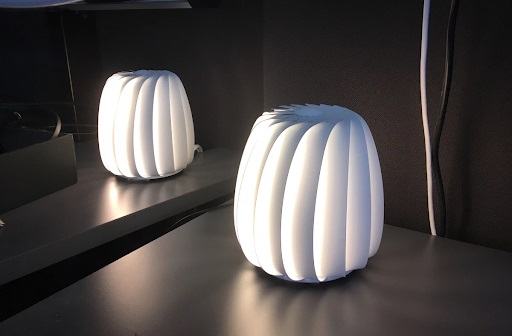
Corti is a specialized little device about the size of a Google Home. However, you won’t find one of these in your living room any time soon.
The tool is currently deploying to emergency response centers worldwide. It listens to medical emergency calls and helps the operator provide the best advice.
It’s most important objective? To identify an incident of cardiac arrest before the humans on the line.
Heart attacks kill more people than anything, yet we’re still notoriously bad at picking up on the telltale signs. This lack of awareness can delay intervention in situations where even a few minutes can have a serious impact on the victim’s survival rate. In fact, for each minute that CPR is delayed, the chance of survival drops by up to 10 percent.
This ML device has a proven track record of identifying cardiac arrest faster, with an astonishing accuracy rate of 93 percent — way higher than the 73 percent typical of a human operator. Its widespread use could save thousands of lives.
The machine learning is necessarily handled on-device, rather than connected to a database in the cloud. In life-threatening situations, the operator needs to provide moment-to-moment life-saving advice, regardless of internet hiccups. Privacy concerns also make a web-connected ML device a little tricky in medical situations.
Corti isn’t just a one-trick pony; its focus is being expanded to include drug overdose and stroke diagnoses, using techniques like vocal analysis.
Corti is powered by the NVIDIA TX2: Arm v8 (64-bit) dual-core + Cortex-A57 quad-core (64-bit).
A More Familiar Focus
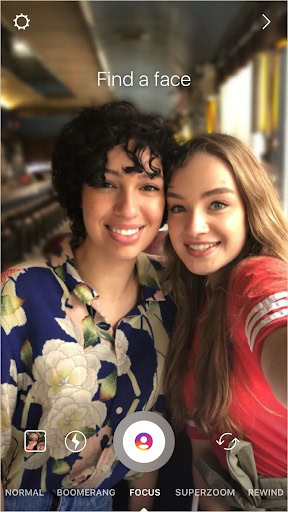
If that use of machine learning got your heart racing a bit too much, here’s a more social palate cleanser.
In 2018, Instagram started rolling out its Focus capability, which lets users create professionally focused selfies and shots that identify faces and blur out the background.
While it’s not exactly stopping heart attacks, this feature offers an intuitive and familiar experience, and it’s possible with the hardware and software improvements that come with machine learning.
Whether using selfie mode, or the standard, back-facing camera, Focus uses the image segmentation network to automatically hone in on the subject of the image while blurring the background to create a professional-looking shot. As you might imagine, this is a complex technique that requires significant additional processing to run quickly and efficiently, and as a result was deployed selectively to higher-end platforms supporting the necessary optimizations. And, due to a powerful collaboration with Arm and the Compute Library team, this also includes a number of devices with Arm Mali GPUs.
So what’s next?
In 2019, companies like Arm will be bolstering devices across the globe with increasing machine learning abilities. We can expect improvements across almost every industry, from precisely targeted pest control in agriculture to more advanced features for autonomous vehicles. Your smart devices will likely get better at tasks like speech recognition, with an increased ability to detect things like inflection and tone.
Keep an eye on Arm if you want to see where on-device machine learning is headed in 2019. With a hockey-stick trend in machine learning capabilities, it will be an exciting year.